
The Leaderboard Illusion

“When a measure becomes a target, it ceases to be a good measure.”
— Charles A. E. Goodhart
Chatbot Arena has become the go-to platform for tracking AI progress, but its current setup risks rewarding leaderboard gaming over real innovation.
Our new study uncovers hidden dynamics that distort rankings — and offers concrete steps to make evaluation fairer, more transparent, and more meaningful for the field.


The Chatbot Arena
Chatbot Arena pits the world's most advanced AI models against each other in a competition driven by human feedback. Leading models enter the arena where they're evaluated through direct comparisons.
Visitors submit their own prompts and questions, receiving responses from two randomly selected models. They then choose their preferred answer in blind evaluations, not knowing which model generated which response. This approach has made Chatbot Arena known for testing AI in authentic, real-world scenarios.

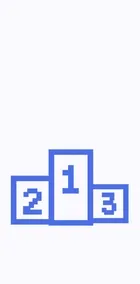
Chatbot Arena, a popular community-driven platform, has become the main battleground for ranking AI models. But as its influence grows, so do concerns about how fairly progress is being measured. We analyzed 2 million battles and 243 models across 42 providers from Jan 2024 to April 2025.
We found that private testing and oversampling give some models an unfair edge, leading to overindexing on Arena-specific metrics rather than real progress. We propose concrete recommendations to make Arena’s evaluation fairer, more transparent, and more reflective of true AI advancements.

Our Findings Reveal Systematic Issues in the Leaderboard

The number of privately tested models per provider based on randomly scraped samples from Arena between January–March, 2025.
Private testing and retraction
Chatbot Arena has an unstated policy of allowing select providers to test many submissions in parallel. We show that certain model developers have benefited from extensive private testing. In a single month, we observe as many as 27 models from Meta being tested privately on Chatbot Arena. Notably, we find that Chatbot Arena does not require all submitted models to be made public, and there is no guarantee that the version appearing on the public leaderboard matches the publicly available API. We show that this skews Arena scores upwards and allows providers that engage with extensive testing to gamify the leaderboard.

Systemic Data Asymmetry Favors Proprietary Models
Proprietary model providers dominate the battles and, as a result, get access to 62.8% of the Chatbot Arena’s data. In comparison, the top 4 academic/nonprofit labs collectively hold less than 1%, a 68:1 disparity. This imbalance stems from hidden testing and uneven model exposure in battles. Chatbot Arena data access drives significant performance gains. The differences in data access between providers matter; we estimate that by training on Chatbot Arena data, model ranking can be improved significantly. In a controlled experimental setting, we observe that increasing the arena training data proportion from (0% → 70%) more than doubles the win-rates from 23.5% to 49.9% on ArenaHard
Disparities in sampling rates enable data asymmetries

Our work finds large biases towards proprietary models in sampling rates. For example, we observe a maximum daily sampling rate of models from a handful of proprietary model of up to 34%, which is 10 times more than what is observed as the maximum sampling from academic providers.

Recommendations

1. Prohibit post-submission retraction
Leaderboard fairness hinges on showing every model’s performance—not just the best attempts.
Granting some providers more chances to private test their models before going public with the best one may lead to inflated rankings.
This lack of transparency makes it hard to distinguish between real progress and strategic optimization. To ensure fairness, we propose that all submissions be final and that providers disclose all their attempts, so the focus remains on fair comparisons and meaningful advancement and not statistical shortcuts.

2. Limit number of variants
Private testing is useful for allowing the community to evaluate models in development while keeping them anonymous before release. However, some providers are testing far more models than others, which may unfairly benefit certain companies and skew the results.
To ensure fairness, we recommend setting a strict and publicly disclosed limit on the number of private models each provider can test at once (e.g., a maximum of 3 models per provider). This prevents excessive testing that could distort the leaderboard and ensures transparent, fair benchmarking for everyone.

3. Ensure models are removed equally
Instead of vague rules, remove models based on clear, performance-driven criteria and fairness. We recommend keeping the leaderboard balanced and fair by removing the weakest 30% of models within each category (proprietary, open-source, open-weight). This ensures no single group (like big tech companies) dominates the rankings and keeps model rankings reliable and meaningful.
Think of it like pruning a garden: trim the least healthy plants in each section to let everything else thrive—without accidentally favoring one type over another. Clear rules = fairer competition.

4. Implement fair sampling
We propose that Arena use the "active sampling" strategy, which prioritizes under-evaluated or high-uncertainty model pairs to reduce statistical bias. Instead of giving every model an arbitrary and disproportionate share, this method focuses on reducing uncertainty in rankings. Imagine trying to rank athletes fairly: you’d prioritize matchups where the outcome is unclear (e.g., a rookie vs. a veteran) to learn faster who’s truly better.
These steps would transform rankings from a resource contest into a true measure of innovation, ensuring all models compete on skill, not just budget or luck.

5. Make model removals transparent
Instead of removing models without notifying the providers, we recommend maintaining a comprehensive list of all models removed from the leaderboard. This will help establish transparency and help ensure that the model removal policy is implemented fairly.
Looking to the Future
AI benchmarking platforms should serve as neutral arbiters of progress, empowering the entire community to measure and improve AI responsibly. Yet current practices—like opaque testing rules and unchecked corporate advantages—risk turning this leaderboard into gatekeepers of inequality rather than engines of innovation.
Adopting our recommendations would align the leaderboard with its research-backed ideals, ensuring rankings reflect genuine progress rather than resource advantages. This transformation is critical to ensure the system upholds its mission of equitable progress for the entire AI community, not just a select few.
Collaborators
This work represents a cross-institutional collaboration with researchers from the following institutions.






Authors and Additional Contributors
Shivalika Singh, Yiyang Nan, Alex Wang, Daniel D’Souza, Sayash Kapoor, Ahmet Üstün, Sanmi Koyejo, Yuntian Deng, Shayne Longpre, Noah A. Smith, Beyza Ermis, Marzieh Fadaee, Sara Hooker, Madeline Smith, Thomas Euyang, Brittawnya Prince, Jenna Cook, Kyle Lastovica, Julia Kligman, Nick Frosst.

Dive into the research
Our research dives into the mechanics of LMArena, uncovering systemic issues through rigorous analysis, theoretical insights, and simulation experiments. We expose flaws in the leaderboard and offer recommendations to improve its reliability.